Data for Analytics
This post from 2022 talks about the need to have better data governance in order to create better data insights.
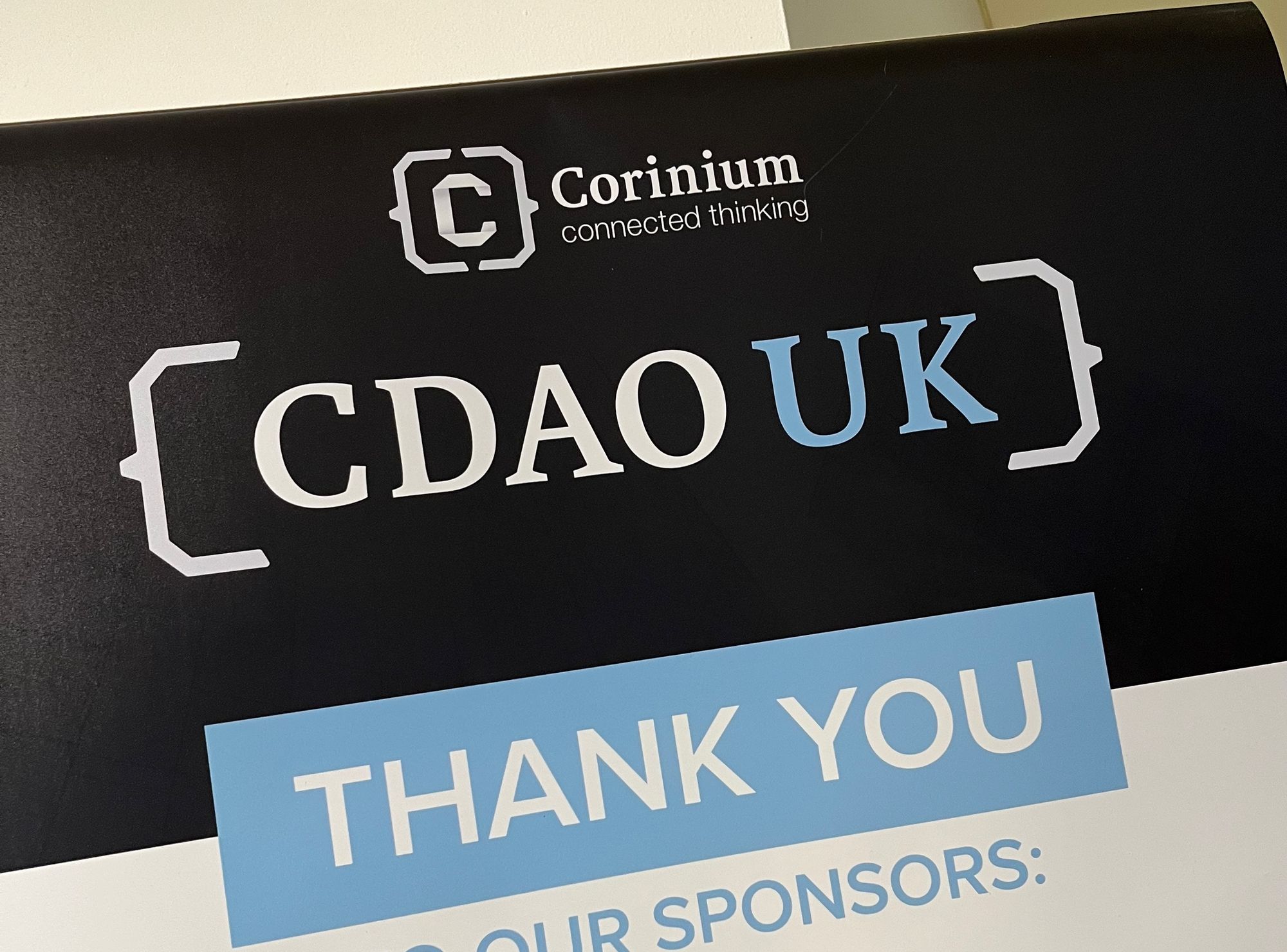
(This post was written in May 2022)
Today I attended the CDAO (Chief Data and Analytics Officer) conference (#CDAOUK) run by Corinium Global Intelligence. It was a great event, with many of the sessions moderated by the excellent Matthew Fryer.
For the past few years, the focus of these conferences tended to be "how to we start doing data science, how do we use AI / ML tools, how do we recruit more data scientists". These questions used to dominate the agenda. People were talking about specific pilot use cases that they had trialled and the headline benefits from that use case. This use case driven approach drove many agile data architectures, focused on a relatively small subset of data attributes. In short, the A in CDAO was the main discussion topic.
It seems that the "conversation" has now become more balanced. Scaling the benefits of data science beyond those first initial use cases has now hit upon some of the challenges that we have been facing into for a number of years. The "How Do I do Data Science" has been balanced by the "How Do I get Data to do Data Science".
Some of the topics that we covered today included:
- How do I get good quality data? (Hint, its about good data governance and data ownership);
- How do I understand the data that I'm presented with? (Hint, its about data catalogs, architecture and design);
- How do I actually get the data into my AI model? (Hint, its about having a good data platform and strategy to bring all data together in a suitable format for analytics) and
- How do I know what I can use my data science for? (Hint, its about data ethics and governance).
It's great that we are talking about all of the things that we need to do to turn our data into business benefit. Having a solid data strategy is key to bringing high quality, well understood data together and maintaining it for sustainable data science and customer benefit.
I'm reminded about some of the things that we did at the Co-op around data ethics, scaling the data platform for data science and moving to the cloud. One of the panels also talked about how as organisations grow in size, they tend to be slowed down legacy systems which are difficult to modernise and catalog. You can also read about how we used Datometry to virtualise our system and replatform in the cloud.